트러스트 알고리즘 (Trust Algorithm, TA)
NIST Special Publication 800-207: Zero Trust Architecture | NIST, https://www.nist.gov/publications/zero-trust-architecture
3.3 트러스트 알고리즘 (신뢰 알고리즘)
ZTA(Zero Trust Architecture)를 배포한 기업의 경우, 정책 엔진(Policy Engine, PE)은 두뇌로, PE의 트러스트 알고리즘(Trust Algorithm, TA)은 기본(primary) 사고 프로세스로 간주될 수 있습니다. TA는 PE가 궁극적으로 리소스에 대한 접근을 승인하거나 거부하기 위해 사용하는 프로세스입니다. PE는 여러 소스(sources)로부터 입력(Input)을 받습니다 (Section 3 참고). 이는 주체, 주체 속성 및 역할, 과거 주체 행동 패턴, 위협 인텔리전스(Threat Intelligence, TI) 소스, 기타 메타데이터 소스에 대한 관찰 가능한 정보가 있는 정책 데이터베이스입니다. 프로세스는 광범위한 범주로 묶일 수 있고 Figure 7에 시각화될 수 있습니다.
그림에서, TA에 제공하는 것에 따라 입력을 범주로 나눌 수 있습니다.
- 접근 요청 (Access Request): 주체의 실제 요청입니다. 요청된 리소스가 기본(primary) 정보로 사용되지만, 요청자에 대한 정보도 사용됩니다. 여기에는 OS 버전, 사용된 소프웨어(예: 요청하는 애플리케이션이 승인된 애플리케이션 목록에 나타납니까?), 패치 수준을 포함할 수 있습니다. 이러한 요소들과 자산 보안 상태(asset security posture)에 따라, 자산에 대한 접근이 제한되거나 거부될 수 있습니다.
- 주체 데이터베이스 (Subject Database): 리소스에 접근을 요청하는 "누구"입니다 [SP800-63]. 이는 기업이나 공동작업자(collaborator)의 주체(사람과 프로세스) 집합이며 할당된 주체 속성/권한 모음입니다. 이 주체와 속성은 리소스 접근을 위한 정책의 기초를 형성합니다 [SP800-162] [NISTIR 7987]. 사용자 식별은 논리적인 식별(예: 계정 ID)과 정책 집행 포인트(Policy Enforcement Point, PEP)가 수행한 인증의 결과를 섞어서 포함할 수 있습니다. 신뢰도를 도출하는 데 영향을 끼칠 수 있는 식별 속성에는 시간과 지리적 위치가 포함됩니다. 여러 주체에 주어진 권한(privileges) 모음은 역할(role)로 간주될 수 있지만, 권한은 그것들이 조직 내 특정 역할에 맞을 수 있다는 단순한 이유 때문이 아니라 개별적인 기준으로 주체에 할당되어야 합니다. 이 모음은 ID 관리 시스템과 정책 데이터베이스에 인코딩되어 저장되어야 합니다. 또, 몇몇 (TA) 변형에서는 과거에 관찰된 주체 행동에 대한 데이터를 여기에 포함할 수도 있습니다 (Section 3.3.1 참고).
- 자산 데이터베이스 (및 관찰 가능한 상태) (Asset Database and Obersavable Status): 각 기업(과 아마도 알려진 비기업/BYOD) 소유의 (어느 정도 물리적이고 가상적인) 자산의 알려진 상태를 포함하는 데이터베이스입니다. 이는 요청을 하는 자산의 관찰 가능한 상태에 비유되며, OS 버전, software present, 그것의 무결성과 위치(네트워크 위치 및 지리적 위치)와 패치 수준을 포함하는 것이 가능합니다. 이 데이터베이스와 비교되는 자산 상태에 따라, 자산에 대한 접근은 제한되거나 거부될 지도 모릅니다.
- 리소스 요구사항 (Resource Requirements): 이 정책 집합은 user ID와 속성 데이터베이스를 보완하고 [SP800-63] 리소스에 대한 접근을 위한 최소 요구사항을 정의합니다. 요구사항은 MFA(Multi-Factor Authentication) 네트워크 위치(예: 해외 IP 주소로부터의 접근 거부), 데이터 민감도, 자산 설정(configuration)을 위한 요청과 같은 인증자 보증 수준을 포함할 수도 있습니다. 이 요구사항들은 데이터 관리인(예: 데이터 책임자)과 데이터를 활용하는 비즈니스 프로세스를 위한 책임자(예: 임무 책임자) 둘 다에 의해 개발되어야 합니다.
- 위협 인텔리전스 (Threat Intelligence): 인터넷 상에서 작동하는 일반적인 위협과 활성 멀웨어에 대한 정보 공유(feed) 혹은 정보를 공유하는 행위(feeds)이다. 이는 (가능한 멀웨어 명령과 제어 노드를 위한 쿼리와 같이) 의심할 수 있는 장치에서 볼 수 있는 통신(communication)에 대한 특정 정보 또한 포함할 수 있습니다. 이러한 정보 공유는 외부 서비스나 내부 스캔 및 발견일 수 있으며 공격 서명 및 완화를 포함 가능합니다. 이는 기업보다 오히려 서비스의 제어 아래에 있을 가능성이 가장 높은 유일한 구성 요소입니다.
각 데이터 소스를 위한 중요도 가중치는 독점 알고리즘(proprietary algorithm)일 수 있거나 기업에 의해 설정될(configure) 수도 있다. 이러한 가중치는 기업에 대한 데이터 소스의 중요도를 반영하는 데 사용할 수 있습니다.
그런 다음 최종 결정은 실행을 위해 정책 관리자(Policy Administrator, PA)로 전달됩니다. PA의 일은 허가된 통신(authorized communication)을 가능하게 하기 위해 필수 PEP를 설정하는 것입니다. ZTA가 어떻게 배포되는지에 따라, 인증(authentication) 결과와 연결 설정 정보(connection configuration information) 를 게이트웨이 및 에이전트 혹은 리소스 포탈로 보내는 것이 포함될 수 있습니다. PA는 또한 정책 요구사항에 따라서 연결을 재인증 및 재허가하기 위해 통신 세션을 보류(hold)하거나 일시중지(pause)할 수도 있습니다. PA는 또한 정책에 기반하여 (예: 타임아웃 이후, 워크플로우가 완료된 경우, 보안 경고 때문에) 연결을 종료하는 명령을 내릴 책임이 있습니다.
3.3.1 TA 변형(Variations)
TA를 구현하는 각양각색의 방법이 있습니다. 각양각색의 구현자는 위 요소의 인지된 중요도에 따라 요소를 저울질하기 바랄 수 있습니다. TA를 구분짓는 데 사용될 수 있는 두 가지 서로 다른 주요 특성이 있습니다. 첫 번째는 이진 결정(binary decisions)이든 전체 "점수(score)"의 가중치를 준 부분이든 신뢰도(confidence level)이든 요소를 평가하는 방법입니다. 두 번째는 동일한 주체나 애플리케이션/서비스나 장치에 의한 다른 요청과 관련하여 요청을 평가하는 방법입니다.
- 기준 기반 vs 점수 기반 (Criteria- Versus Score-Based): 기준 기반 TA는 리소스에 접근이 승인되거나 작업(예: 읽기/쓰기)이 허용되기 전에 충족되어야만 하는 적격의 속성 집합을 가정합니다. 이 기준은 기업에 의해 설정되며 모든 리소스에 대해 독립적으로 설정되어야 합니다. 모든 기준이 충족되어야만 접근이 승인되거나 리소스에 작업이 적용됩니다. 점수 기반 TA는 모든 데이터 소스에 대한 값과 기업에서 설정한 가중치를 기반으로 신뢰도를 계산합니다. 설정된 리소스 임계값보다 점수가 더 크면, 접근이 허용되거나 작업이 수행됩니다. 그렇지 않으면, 요청이 거부되거나 접근 권한이 감소합니다 (예: 파일에 대해 읽기 접근은 승인되지만 쓰기 권한은 아님).
- 단독형 vs 맥락형 (Singular Versus Contextual): 단독형 TA는 각 요청을 개별적으로 취급하며 평가 시 주체 내역(subject history)을 고려하지 않습니다. 이는 더 빠른 평가가 가능하지만, 공격이 주체의 허용된 역할 내에 있다면 탐지하지 못할 위험이 있습니다. 맥락형 TA는 접근 요청을 평가할 때 주체나 네트워크 에이전트의 최근 내역을 고려합니다. 이는 PE가 모든 주체와 애플리케이션 상에서 몇몇 상태 정보를 유지해야 하지만, 주어진 주체에 대해 PE가 보는 것과 어울리지 않는 패턴 안에서 정보에 접근하기 위해 변조된 자격증명을 사용하는 공격자를 탐지할 가능성이 더 클 수 있다는 것을 의미합니다. 또한, 주체가 통신할 때 상호작용하는 PA(와 PEP)가 사용자 행위를 PE에게 알려야 함을 의미합니다. 주체 행위 분석은 수용 가능한 사용 모델을 제공하기 위해 사용할 수 있으며, 이 행위에서 벗어나면 추가적인 인증을 확인하거나 리소스 요청이 거부될 수 있습니다.
두 요소가 항상 서로 의존성이 있는 것은 아닙니다. 모든 주체 및/또는 장치에 신뢰도를 할당하며 여전히 모든 접근 요청을 독립적으로(즉, 단독형) 고려하는 TA를 가지는 것이 가능합니다. 그러나 맥락형 점수 기반 TA는 점수가 요청 계정에 대해 현재 신뢰도를 제공하고 인간 관리자에 의해 수정된 정적 정책보다 변화하는 요소에 더 빠르게 적응하기 때문에, 보다 동적이고 세분화된 접근 제어 기능을 제공할 것입니다.
이상적으로, ZTA 트러스트 알고리즘은 맥락형이어야 하지만, 기업이 이용할 수 있는 인프라 구성 요소에서는 이것이 항상 가능하지 않을 수 있습니다. 맥락형 TA는 공격자가 해킹된 주체 계정 혹은 내부자 공격을 위해 "평범한" 접근 요청 집합에 가깝게 머무르는 위협을 완화할 수 있습니다. 트러스트 알고리즘을 정의하고 구현할 때 보안(security), 사용성(usability), 비용효과(cost-effectiveness)를 견주어보는 것이 중요하다. 조직 내에서 그들의 임무 기능 및 역할에 대한 내역의 동향 및 규범과 일치하는 행위에 대해 주체에게 재인증을 지속적으로 요청하는 것은 사용성 문제를 야기할 수 있습니다. 예를 들어, 만약 에이전시의 HR 부서 직원이 일반적인 근무일에 보통 20~30개 직원 기록에 접근하는 경우, 맥락형 TA는 접근 요청이 갑자기 하루에 100개의 기록을 초과하면 경고를 보낼 수 있습니다. 또 맥락형 TA는 만약 누군가 정상적인 업무 시간 이후에 접근 요청을 하고 있다면 경고를 보낼 수도 있습니다. 해킹된 HR 계정으로 기록을 유출시키는 공격자일 수 있기 때문입니다. 이는 맥락형 TA가 공격을 탐지할 수 있는 반면에 단독형 TA는 새로운 행위를 탐지하는 데 실패할 수 있다는 예입니다. 또 다른 예로, 보통 정상적인 업무 시간 동안 금융 시스템에 접근하는 회계사가 이제는 인식할 수 없는 위치에서 한밤중에 시스템에 접근하려 시도하고 있습니다. 맥락형 TA는 경고를 발생시킬 수 있고 주체에게 NIST Special Publication 800-63A [SP800-63A]에 요약된 바와 같이 보다 엄격한 신뢰 수준(confidence level)이나 기타 기준을 충족시키도록 해야 할 수 있습니다.
각 리소스에 대한 일련의 기준이나 가중치/임계값을 개발하려면 계획을 세우고 테스트를 해야 합니다. 기업 관리자는 ZTA의 초기 구현 중 승인되어야 하는 접근 요청들이 잘못된 설정 때문에 거부되는 문제에 직면할 수 있습니다. 이는 배포의 초기 "세부 조정(tuning)" 단계가 시작됩니다. 계속 기업의 업무 프로세스가 기능하도록 허용하면서 정책들이 시행되는 것을 보장하기 위해 기준이나 점수 산정 가중치가 보정될 필요가 있을 수 있습니다. 이 세부 조정 단계가 얼마나 계속될지는 워크플로우에서 사용되는 리소스에 대해 잘못된 접근 거부/승인의 경과(progress) 및 허용 오차(tolerance)를 위해 기업에서 정의한 메트릭스(enterpise-defined metrics)에 달려 있습니다.
3.3 Trust Algorithm
For an enterprise with a ZTA deployment, the policy engine can be thought of as the brain and the PE’s trust algorithm as its primary thought process. The trust algorithm (TA) is the process used by the policy engine to ultimately grant or deny access to a resource. The policy engine takes input from multiple sources (see Section 3): the policy database with observable information about subjects, subject attributes and roles, historical subject behavior patterns, threat intelligence sources, and other metadata sources. The process can be grouped into broad categories and visualized in Figure 7.
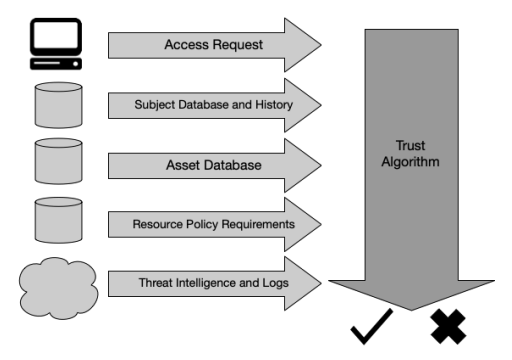
In the figure, the inputs can be broken into categories based on what they provide to the trust algorithm.
• Access request: This is the actual request from the subject. The resource requested is the primary information used, but information about the requester is also used. This can include OS version, software used (e.g., does the requesting application appear on a list of approved applications?), and patch level. Depending on these factors and the asset security posture, access to assets might be restricted or denied.
• Subject database: This is the “who” that is requesting access to a resource [SP800-63]. This is the set of subjects (human and processes) of the enterprise or collaborators and a collection of subject attributes/privileges assigned. These subjects and attributes form the basis of policies for resource access [SP800-162] [NISTIR 7987]. User identities can include a mix of logical identity (e.g., account ID) and results of authentication checks performed by PEPs. Attributes of identity that can be factored into deriving the confidence level include time and geolocation. A collection of privileges given to multiple subjects could be thought of as a role, but privileges should be assigned to a subject on an individual basis and not simply because they may fit into a particular role in the organization. This collection should be encoded and stored in an ID management system and policy database. This may also include data about past observed subject behavior in some (TA) variants (see Section 3.3.1).
• Asset database (and observable status): This is the database that contains the known status of each enterprise-owned (and possibly known nonenterprise/BYOD) asset (physical and virtual, to some extent). This is compared to the observable status of the asset making the request and can include OS version, software present, and its integrity, location (network location and geolocation), and patch level. Depending on the asset state compared with this database, access to assets might be restricted or denied.
• Resource requirements: This set of policies complements the user ID and attributes database [SP800-63] and defines the minimal requirements for access to the resource. Requirements may include authenticator assurance levels, such as MFA network location (e.g., deny access from overseas IP addresses), data sensitivity, and requests for asset configuration. These requirements should be developed by both the data custodian (i.e., those responsible for the data) and those responsible for the business processes that utilize the data (i.e., those responsible for the mission).
• Threat intelligence: This is an information feed or feeds about general threats and active malware operating on the internet. This could also include specific information about communication seen from the device that may be suspect (such as queries for possible malware command and control nodes). These feeds can be external services or internal scans and discoveries and can include attack signatures and mitigations. This is the only component that will most likely be under the control of a service rather than the enterprise.
The weight of importance for each data source may be a proprietary algorithm or may be configured by the enterprise. These weight values can be used to reflect the importance of the data source to an enterprise.
The final determination is then passed to the PA for execution. The PA’s job is to configure the necessary PEPs to enable authorized communication. Depending on how the ZTA is deployed, this may involve sending authentication results and connection configuration information to gateways and agents or resource portals. PAs may also place a hold or pause on a communication session to reauthenticate and reauthorize the connection in accordance with policy requirements. The PA is also responsible for issuing the command to terminate the connection based on policy (e.g., after a time-out, when the workflow has been completed, due to a security alert).
3.3.1 Trust Algorithm Variations
There are different ways to implement a TA. Different implementers may wish to weigh the above factors differently according to the factors’ perceived importance. There are two other major characteristics that can be used to differentiate TAs. The first is how the factors are evaluated, whether as binary decisions or weighted parts of a whole “score” or confidence level. The second is how requests are evaluated in relation to other requests by the same subject, application/service, or device.
• Criteria- versus score-based: A criteria-based TA assumes a set of qualified attributes that must be met before access is granted to a resource or an action (e.g., read/write) is allowed. These criteria are configured by the enterprise and should be independently configured for every resource. Access is granted or an action applied to a resource only if all the criteria are met. A score-based TA computes a confidence level based on values for every data source and enterprise-configured weights. If the score is greater than the configured threshold value for the resource, access is granted, or the action is performed. Otherwise, the request is denied, or access privileges are reduced (e.g., read access is granted but not write access for a file).
• Singular versus contextual: A singular TA treats each request individually and does not take the subject history into consideration when making its evaluation. This can allow faster evaluations, but there is a risk that an attack can go undetected if it stays within a subject’s allowed role. A contextual TA takes the subject or network agent’s recent history into consideration when evaluating access requests. This means the PE must maintain some state information on all subjects and applications but may be more likely to detect an attacker using subverted credentials to access information in a pattern that is atypical of what the PE sees for the given subject. This also means that the PE must be informed of user behavior by the PA (and PEPs) that subjects interact with when communicating. Analysis of subject behavior can be used to provide a model of acceptable use, and deviations from this behavior could trigger additional authentication checks or resource request denials.
The two factors are not always dependent on each other. It is possible to have a TA that assigns a confidence level to every subject and/or device and still considers every access request independently (i.e., singular). However, contextual, score-based TAs would provide the ability to offer more dynamic and granular access control, since the score provides a current confidence level for the requesting account and adapts to changing factors more quickly than static policies modified by human administrators.
Ideally, a ZTA trust algorithm should be contextual, but this may not always be possible with the infrastructure components available to the enterprise. A contextual TA can mitigate threats where an attacker stays close to a “normal” set of access requests for a compromised subject account or insider attack. It is important to balance security, usability, and cost-effectiveness when defining and implementing trust algorithms. Continually prompting a subject for reauthentication against behavior that is consistent with historical trends and norms for their mission function and role within the organization can lead to usability issues. For example, if an employee in the HR department of an agency normally accesses 20 to 30 employee records in a typical workday, a contextual TA may send an alert if the access requests suddenly exceed 100 records in a day. A contextual TA may also send an alert if someone is making access requests after normal business hours as this could be an attacker exfiltrating records by using a compromised HR account. These are examples where a contextual TA can detect an attack whereas a singular TA may fail to detect the new behavior. In another example, an accountant who typically accesses the financial system during normal business hours is now trying to access the system in the middle of the night from an unrecognizable location. A contextual TA may trigger an alert and require the subject to satisfy a more stringent confidence level or other criteria as outlined in NIST Special Publication 800-63A [SP800-63A].
Developing a set of criteria or weights/threshold values for each resource requires planning and testing. Enterprise administrators may encounter issues during the initial implementation of ZTA where access requests that should be approved are denied due to misconfiguration. This will result in an initial “tuning” phase of deployment. Criteria or scoring weights may need to be adjusted to ensure that the policies are enforced while still allowing the enterprise’s business processes to function. How long this tuning phase lasts depends on the enterprise-defined metrics for progress and tolerance for incorrect access denials/approvals for the resources used in the workflow.
- Rose, S. , Borchert, O. , Mitchell, S. and Connelly, S. (2020), Zero Trust Architecture, Special Publication (NIST SP), National Institute of Standards and Technology, Gaithersburg, MD, [online], https://doi.org/10.6028/NIST.SP.800-207, https://tsapps.nist.gov/publication/get_pdf.cfm?pub_id=930420
- 제로트러스트 가이드라인 1.0 전체본 (2023) – KISA, https://www.kisa.or.kr/2060205/form?postSeq=20&page=1#fnPostAttachDownload
'컴퓨터 과학' 카테고리의 다른 글
개체명 인식 (Named-Entity Recognition, NER) (0) | 2018.10.12 |
---|---|
RPC, CORBA, RMI, SOAP, REST 쉬운 설명 (작성 중) (0) | 2017.07.28 |
[데이터 통신] (제1부 개요) 제1장 데이터통신, 데이터 네트워크, 인터넷 (0) | 2016.08.19 |
댓글
이 글 공유하기
다른 글
-
개체명 인식 (Named-Entity Recognition, NER)
개체명 인식 (Named-Entity Recognition, NER)
2018.10.12 -
RPC, CORBA, RMI, SOAP, REST 쉬운 설명 (작성 중)
RPC, CORBA, RMI, SOAP, REST 쉬운 설명 (작성 중)
2017.07.28 -
[데이터 통신] (제1부 개요) 제1장 데이터통신, 데이터 네트워크, 인터넷
[데이터 통신] (제1부 개요) 제1장 데이터통신, 데이터 네트워크, 인터넷
2016.08.19